In our first post in this series, we introduced the concept of AI in lending and examined the first part of my conversation on the subject with ChatGPT – a so-called “large language model” AI that has made recent headlines. After a general introduction to the idea, I asked ChatGPT about the benefits of AI in lending.
In this post I’ll share the next part of my discussion with this AI on the risks of AI in lending.
Remember, again, that this conversation is presented unedited except for formatting.
The Risks of AI in Lending
Query
Let’s move on to the risks you mentioned. Can you talk about how bias can be an issue with AI in lending and provide some specific examples?
Response
Certainly. Bias can be a significant issue when using AI in lending, as it can lead to discriminatory or unfair lending practices. AI systems are only as unbiased as the data they are trained on, and if the training data contains biases, the AI system may learn and perpetuate these biases in its predictions and decisions.
For example, if an AI system is trained on data that reflects historical lending patterns, it may be biased against certain groups, such as women or minorities, who have been traditionally underserved by the lending industry. This can result in discriminatory lending practices, where certain groups are unfairly denied access to credit or offered loan terms that are less favorable than those offered to others.
Another example of bias in AI lending is when an AI system is trained on data that is unrepresentative of the population it is being used to serve. For example, if an AI system is trained on data from a predominantly urban area, it may not accurately predict the creditworthiness of borrowers from rural areas.
It is important for lenders to be aware of these potential biases in AI and to take steps to mitigate them, such as using diverse training data and regularly auditing their AI systems for biases. This can help to ensure that AI systems are used in an ethical and fair manner and do not perpetuate discriminatory lending practices.
Query
Next, can you talk about steps that can be taken to increase the transparency of AI lending decisions?
Response
Yes, transparency is an important aspect of responsible AI use, especially in the context of lending. Here are some steps that can be taken to increase the transparency of AI lending decisions:
- Explanation of decision-making processes: AI systems should be designed to provide clear and transparent explanations of how they make lending decisions, including which data inputs and algorithms were used. This can help to increase understanding and trust in the AI system among lenders and borrowers.
- Data auditability: The data used to train and operate AI systems should be auditable and subject to ongoing review to ensure that it is accurate, relevant, and free of bias. This can help to increase transparency and accountability in the lending process.
- Regular performance evaluation: AI systems should be regularly evaluated to ensure that they are performing as intended and are not making discriminatory or unfair lending decisions. This can include regular performance audits, testing with diverse data sets, and monitoring for unusual patterns or outliers in the data.
- Collaboration with stakeholders: AI lenders should work closely with stakeholders, including regulators, industry groups, and customer organizations, to ensure that their AI systems are transparent, fair, and aligned with industry standards and best practices.
By taking these steps to increase transparency, AI lenders can help to build trust and confidence in their systems and ensure that they are used in a responsible and ethical manner.
Query
Can you discuss how AI could be misused, intentionally or unintentionally, in lending?
Response
Yes, AI systems can be misused in lending in several ways, both intentionally and unintentionally. Some potential examples include:
- Discriminatory lending practices: AI systems can be designed or trained in a way that results in discriminatory lending practices, such as unfairly denying loans to certain groups based on factors such as race, gender, or age.
- Privacy violations: AI systems may collect and use sensitive personal information in the lending process, and this information could be misused or exposed in data breaches. This can result in privacy violations and harm to borrowers.
- Unfair loan terms: AI systems may offer loan terms that are unfair or exploitative, such as high-interest rates or hidden fees. This can result in financial harm to borrowers and undermine trust in the lending industry.
- Unjustified rejections: AI systems may reject loan applications without a clear explanation of why the application was rejected, leaving borrowers without access to credit and without a clear understanding of what they need to do to improve their chances of approval in the future.
It is important for lenders to be aware of these potential risks and to take steps to mitigate them, such as using transparent and auditable AI systems, regularly monitoring performance and outcomes, and working with stakeholders to ensure that AI is used in a responsible and ethical manner.
Summary of the Risks of AI in Lending
In this part of my discussion with ChatGPT, we covered 3 major risk areas to be aware of when considering and implementing AI technology in the lending process. This list should not be considered comprehensive, but it does give a great overview of the major risk categories to address.
Of the utmost importance is understanding that the AI “model” (that is, the code and data that your AI uses to make decisions based on how it’s been trained) is only as good – as unbiased, as accurate in assessing credit worthiness, etc. – as the data used to train it.
If you train a model with data that reflects bias against protected groups, the model will be biased against protected groups.
As part of addressing this issue, transparency around all the components and processes involving AI in the lending pipeline is key. Therefore a lack of transparency is a key risk factor to be aware of at all stages.
Finally, we discussed how AI can be misused – even unintentionally – in the lending process with examples ranging from blatant discrimination to fuzzy logic behind application denials.
No matter how a bank approaches the use of AI in its systems (including deciding NOT to use AI at all), there are both profound benefits and profound risks to be aware of.
So how should a bank proceed?
In my next and final post about my conversation with ChatGPT, I’ll share how this AI suggested a bank go about deciding how to implement AI in its lending. We cover whether the bank should build such tools internally and what to consider if they do, then we discuss hiring a third party firm or service provider and what to consider in that situation.
To follow along and catch the conclusion of this series on AI in lending, be sure to subscribe below.
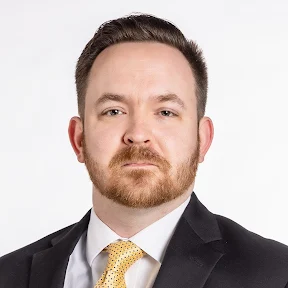
John is the Chief Technology Officer for Premier Insights, Inc. and Radiant Lending Technologies LLC.